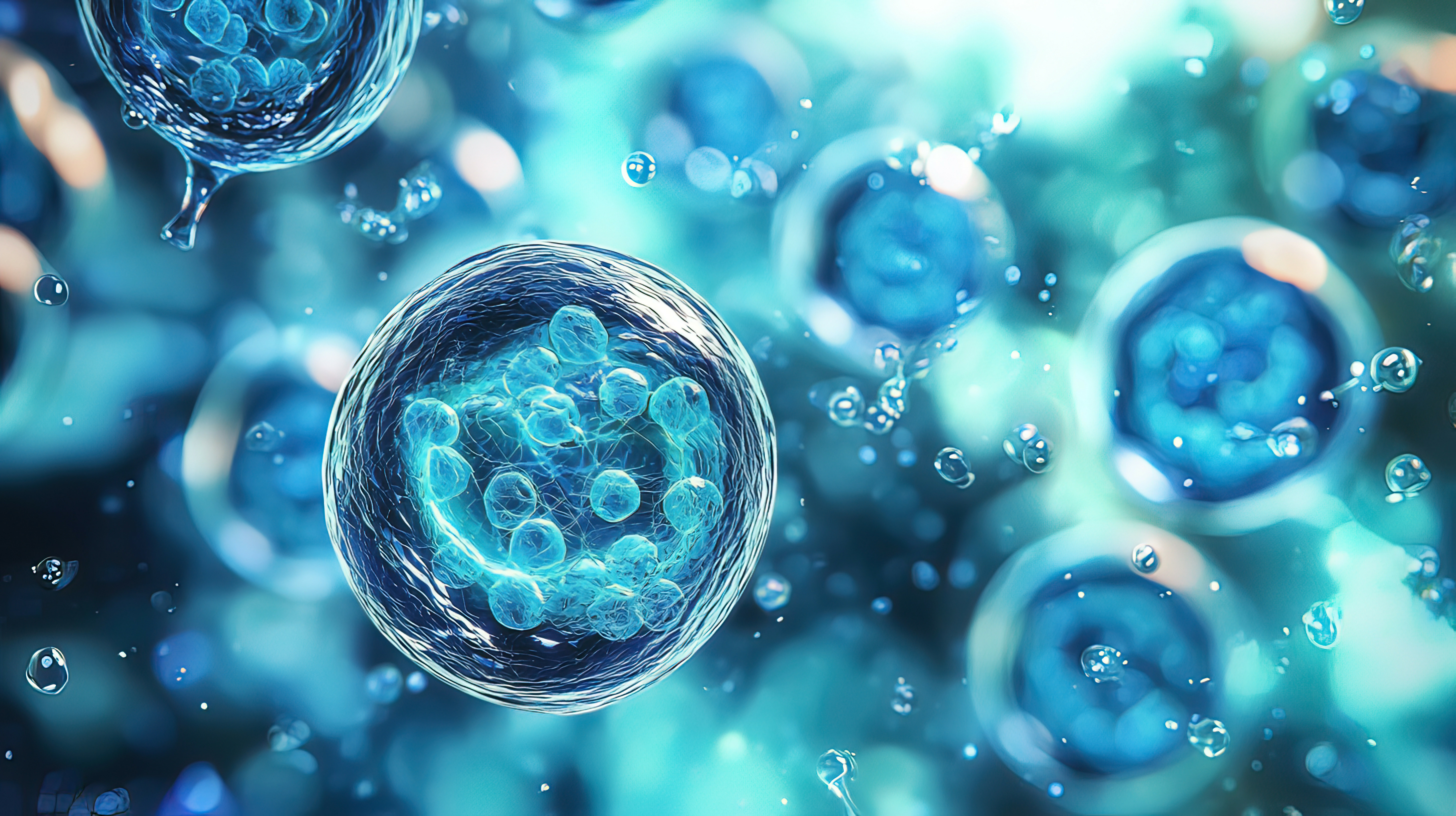
Expression constructs are among the most widely used tools in modern biological research. These constructs allow a gene of interest to be introduced into a host cell, where the cell's transcriptional and translational machinery is harnessed to synthesize the protein encoded by the gene. The applications of expression constructs are vast and varied. For example, expression constructs have been used to express recombinant human insulin for the treatment of diabetes since 1982. Today, expression constructs can be used to study gene function, create transgenic organisms, or even treat genetic diseases in gene therapy.
Traditionally, expression constructs have been generated by assembling naturally occurring DNA sequences. A cDNA derived from an endogenous DNA sequence encoding a protein of interest is introduced into an expression vector under the control of a promoter and other regulatory elements that determine when and where the protein will be expressed. These regulatory sequences are typically derived from the host system, allowing host transcription factors to regulate gene expression.
However, recent developments in artificial intelligence (AI) suggest that soon we may no longer need to rely on naturally occurring sequences. In recent years, artificial intelligence (AI) has emerged as a transformative force in biotechnology. AI's ability to analyse vast datasets, identify patterns, and make predictions has opened up new possibilities, such as predicting 3D protein structure or indeed, designing expression constructs.
Cis-regulatory elements (CREs) are key to regulating gene expression, which is crucial for determining the identity and function of every cell in an organism. CREs govern processes ranging from embryonic development to the immune response to infection. These elements can be introduced into expression constructs to control the spatial and temporal expression of a gene of interest.
Researchers from the Yale School of Medicine, The Jackson Laboratory, and the Broad Institute of MIT and Harvard recently unveiled CODA (Computational Optimization of DNA Activity), a platform designed to engineer synthetic CREs that mediate cell-type-specific gene expression. To build this platform, the team trained a convolutional neural network (CNN) on data from massively parallel reporter assays, which quantified the effects of 776,474 different regulatory nucleotide sequences on gene expression across three distinct cell types. The trained neural network could successfully predict the activity of previously unknown CREs.
CODA generates novel CRE sequences and uses the CNN to predict their activity in different cell types. These sequences are then scored based on their ability to differentiate gene expression between target and non-target cells. This score is then used to update the CRE sequence so that the difference in gene expression between the target and non-target cells is maximised in an iterative process. Notably, the synthetic CREs designed by CODA demonstrated superior cell-type specificity compared to naturally occurring CREs in humans.
In this way, synthetic CREs could be used to improve gene therapy by ensuring that therapeutic genes are expressed only in diseased cells, such as malfunctioning neurons in Parkinson’s disease or even cancerous cells, while avoiding expression in healthy cells, where the protein could cause adverse effects.
Whilst using naturally occurring coding sequences guarantees that the correct protein is produced, it may not always represent the most optimal sequence. Multiple mRNA sequences can encode the same protein and with 64 codons for just the 20 most common amino acids, the possible variations are vast. Research in the field of mRNA vaccines has demonstrated that mRNA sequence optimisation can improve protein expression, improving the vaccine’s potency and efficacy, as well as making the vaccine more cost-effective. However, the sheer number of potential mRNA sequences makes it impractical to test each one experimentally.
One notable advancement in this field is the Linear Design algorithm, developed by researchers at Baidu USA. This algorithm optimizes mRNA sequences for stability and immunogenicity, specifically for mRNA vaccine design. The researchers used a deterministic finite-state automaton to represent all possible mRNA sequences encoding the target protein. Through lattice parsing, an approach borrowed from computational linguistics, they identified the most stable mRNA sequences from the set of possibilities. In just 11 minutes, Linear Design identified the most stable mRNA sequence for the 1,273-amino-acid COVID-19 spike protein. When injected into a mouse model, this optimized mRNA sequence led to a 128-fold increase in antibody production compared to a benchmark mRNA COVID-19 vaccine.
In 2021, Sanofi recognized the importance of this work and obtained an exclusive license for Baidu USA’s Linear Design patent, with plans to use it in the development of future mRNA-based vaccines and therapeutics.
Another approach to mRNA optimization is the CodonBERT algorithm, which modifies Google’s BERT (Bidirectional Encoder Representations from Transformers) model to understand the "language" of mRNA. CodonBERT was trained on 10 million mRNA sequences to identify taxonomic relationships between mRNA sequences and to identify missing codons. By utilizing the transformer architecture (the same architecture that brought you chatGPT), CodonBERT can “read” mRNA sequences in both directions, allowing for richer context when performing these tasks. When extended to perform specific mRNA prediction tasks, CodonBERT could accurately predict mRNA sequences that would lead to higher gene expression or reduced mRNA degradation.
In a similar vein, GEMORNA, a generative AI model developed by Raina Biosciences, builds upon transformer-based architecture to generate complete mRNA sequences, including untranslated regions (UTRs). UTRs have an important role in controlling translation and the stability of the mRNA. GEMORNA is the first model of its kind to generate full mRNA sequences that include these UTRs. mRNAs incorporating GEMORNA designed 5’ UTRs exhibited greater mean ribosome load than naturally occurring 5’ UTRs, suggesting that the AI-designed sequences would improve protein expression.
In eukaryotic genes, introns are non-coding sequences that are spliced out of mRNA. Historically, they have been considered the ‘junk’ in the genome and, partially due to their large size, they have been omitted from expression constructs in eukaryotic host cells.
However, in 2021, Dr. Kärt Tomberg demonstrated that adding artificial introns to a viral DNA sequence for the COVID-19 spike protein led to nearly a ten-fold increase in protein production in eukaryotic host cells1. This discovery prompted Tomberg to co-found the company Expression Edits with Dr. Allan Bradley, a company focused on enhancing gene expression in expression constructs by introducing introns. They have recently announced that they have developed the Genetic Syntax Engine, which uses AI to identify optimal sites for intron insertion and to design the corresponding intron sequences.
Recently, Boehringer Ingelheim partnered with ExpressionEdits and obtained an exclusive license for the Genetic Syntax Engine platform. This collaboration aims to accelerate the development of high-impact gene therapies, leveraging AI to improve the efficiency of gene expression.
AI is rapidly transforming the design of expression constructs. From optimising regulatory elements and mRNA sequences to incorporating introns for enhanced protein production, these innovations are pushing the boundaries of what is possible in genetic research and medicine. As AI-driven platforms continue to evolve, the future of gene therapy and synthetic biology holds exciting potential for treating a wide range of diseases and advancing our understanding of genetics.
There are also exciting opportunities for patentability. Advances in AI platforms that provide real world benefits by improving the design of expression vectors, including the examples discussed here, can be protected using patents. CREs or intron sequences would normally face difficulties in patentability as they would inevitably have been derived from naturally occurring nucleotide sequences, especially with our limited understanding of the genetic language of non-coding sequences. The existence of prior art disclosing sequences with high sequence identity would require the scope of any patent application to be narrowed. However, this may no longer be an issue with AI’s assistance in designing sequences with little similarity to anything that has come before. Additionally, protecting aspects of a platform may be advantageous when multiple sequence possibilities exist that could achieve similar benefits but cannot be easily capture by a single common sequence feature.
Isabelle is a trainee patent attorney working in the Life Sciences team. Isabelle has a degree in Biological Natural Sciences from the University of Cambridge, with an integrated MSci in Systems Biology. As part of her master’s degree, she investigated the regulation of tRNA gene expression during neuronal development and degeneration.
Email: Isabelle.Murray@mewburn.com
Our IP specialists work at all stage of the IP life cycle and provide strategic advice about patent, trade mark and registered designs, as well as any IP-related disputes and legal and commercial requirements.
Our peopleWe have an easily-accessible office in central London, as well as a number of regional offices throughout the UK and an office in Munich, Germany. We’d love to hear from you, so please get in touch.
Get in touch