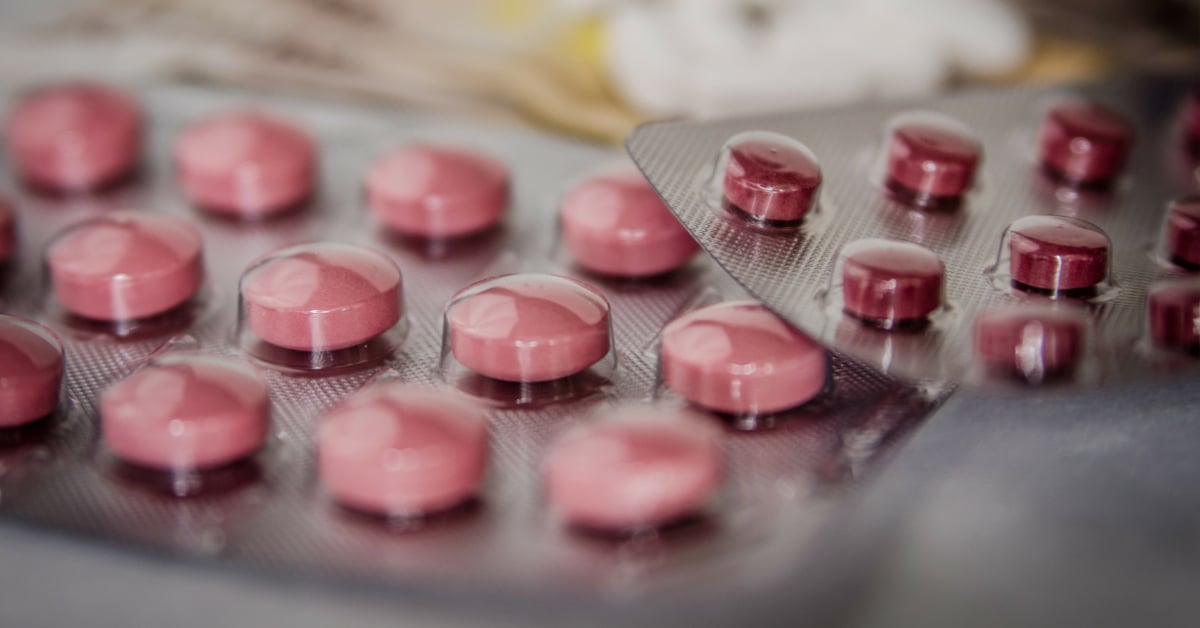
Algorithms (sometimes collectively referred to as ‘digital health’) are changing the way that healthcare is practised, from diagnostics to monitoring and the determination of a course of treatment. The number of algorithms available dealing with an aspect of health has increased dramatically in the last few years, ranging from apps on personal computing devices to specialised platforms for doctors and hospitals. These new tools come with new challenges, some of which we’ll touch on below.
Algorithms as medical devices challenge many existing regulatory systems. First, the explosion in the number of digital health products and entities creating these products means that new people are exposed to medical devices regulations, which previously mostly concerned large pharmaceutical and medical devices companies well versed in dealing with these. These new actors may be much less familiar with the system and may be forced to interact with regulatory bodies that suffer from a shortage of skills necessary to properly assess the new technologies.
Second, as mentioned above many digital health products blur the line between healthcare and wellbeing/lifestyle. Where a product falls on this line often has strong implications from a regulatory perspective, with healthcare being much more tightly regulated than wellbeing. The question of what qualifies as a medical device is defined by statutes in the EU and in the US, as interpreted by case law. These take into account, in various ways and to various degrees, the intended purpose of the device and the risk associated with this. Flexibility (to enable the law to apply to evolving technology) and clarity in this assessment (where, as explained above, “clarity” may need to be assessed from a different point of view than previously to enable new actors to identify the standards that apply to them) seem crucial.
Third, machine learning (ML) as a medical device may come with specific challenges in relation to transparency and stability, which current medical devices regulations often do not explicitly address. Some ML algorithms work as so-called “black boxes”, such that their internal workings are either unknown or not interpretable to us. This can have an impact on how safety and effectiveness of the medical device are assessed. Indeed, interpretability of a model may help to support a credible scientific case for the tool integrating the model – for example to give credibility for an identified relationship between a marker and a condition being causal rather than a correlation.
Further, some ML algorithms are dynamic in the sense that they constantly retrain as they gain access to more data. Whether and to what extent each new update should be re-evaluated can have a very large impact on both risk to the population and burden on the manufacturer. Balancing these is essential in order to maintain trust from the population and avoid deterring manufacturer from providing improved products. The FDA is actively looking at these issues and has proposed, in a discussion paper available here, “a total product lifecycle-based regulatory framework for [AI and ML] technologies that would allow for modifications to be made from real-world learning and adaptation, while still ensuring that the safety and effectiveness of the software as a medical device is maintained”. This was open to comments, but the results of the consultation are not yet available at the time of writing.
Data sharing and privacy is another crucial point to be considered in this ecosystem. Many digital health devices are data hungry, and also produce heaps of data that is often of a personal and sensitive nature. This includes genomic data, physiological readings, medical images, family history, health and nutrition habits, etc. Broadly speaking, making data available enables the development of better tools, and ultimately improves healthcare. Conversely, sub-optimal sharing in a healthcare system may result in variations in the quality of testing services (as this may depend on the data that is available), potential misdiagnosis, and avoidable delays in patient diagnosis. For example, inadequate sharing of genomic data and disease associations within a healthcare system may mean that a patient will not be diagnosed as being at risk for a certain disease because this information simply is not available (or not available as information supported by sufficient evidence) to the centre where the patient was tested.
In many national health systems, an infrastructure for efficiently and safely sharing medically relevant data is lacking. Building such an infrastructure requires tremendous curation and standardisation efforts. The provision of such infrastructures by private companies, while representing a solution to a very real problem, does not come without obvious risks. Further, any sharing of such data will have to be accompanied with a careful assessment of issues of privacy, security and consent. These are complex issues since medical data, at least when multiple pieces of information can be combined, can often identify an individual (see for example here and here), but links and associations between different pieces of data often cannot be removed without significantly impacting how useful that data is for research. Further, scientists may not be able to foresee what a particular piece of data may be useful for in the future, and as such consent would ideally have to be a dynamic process. Balancing the rights of a person to control their data and the need to not place unreasonable burden on future progress can be difficult. Recognising these issues, the UK government has recently appointed a National Data Guardian for Health and Social Care, with the aim of building public trust for the use of patient data. Meanwhile companies such as Sano Genetics are already providing solutions that attempt to tackle the issue of consent in relation to genomic data.
Current healthcare practices usually involve human intervention in any decision-making process. Automated decision making by algorithms pose new questions such as whether the decision should have to be explained to the user, where the liability lies in case of an erroneous decision, and whether / how the biases and limitations inherent to a system that is trained on data are to be assessed and understood by the user. Additionally, the nature of the user itself may change as apps empower citizens to get more actively involved in their health management (switching from a medical devices market that was primarily B2B to a market that increasingly includes B2C products).
ML/AI scientists will all be familiar with the fact that the output of an algorithm will carry biases associated with the data it was trained on. This problem has recently hit the headlines in relation to facial recognition software, where the reported accuracy of an algorithm may in fact be much lower for some populations that were not sufficiently represented in the training data (e.g. ethnic minorities). Similar problems can occur in healthcare, where e.g. a prognostic algorithm that is trained on data including an over-representation of Caucasian males is likely to perform much better for this demographic than for e.g. African women. Not only is this potentially a problem from a safety point of view, it also potentially poses problems from a discrimination point of view. Indeed, cutting edge healthcare tools may only become available for those demographics for which data exists, and this is likely to be a self-reinforcing phenomenon since these tools will often collect the data that will improve the tools. Human decision is of course not free of biases, but the perceived “objectivity” of machine decisions and the complexity of the processes through which they can be biased might make biases harder to spot, and harder for us to “swallow” as a society.
Currently, machine learning assists but does not replace the physician, such that ultimately the buck still stops with the trained practitioner making the decision in a more or less conventional manner. However, we do have to ask ourselves whether this will remain appropriate in a world where physicians are expected to increasingly rely on technology which they may not understand the ins-and-outs of (or even have access to that information). Conversely, if we fail to embrace these technologies, whether by enabling physicians to safely rely on them, or by providing a framework in which we can do away with human intervention altogether, are we denying millions of people better healthcare?
As mentioned above, many governments and institutions (including the phg foundation, which has produced much fascinating reading material about many of the points mentioned above) are devoting resources to tackling these questions. Digital health technology is not a future, it’s a reality. It’s a reality that will affect us all, and it is important for us all to think and talk now about what we want our reality to look like, while these discussions are ongoing.
Camille is a Partner and Patent Attorney at Mewburn Ellis. She does patent work in the life sciences sector, with a particular focus on bioinformatics/computational biology, precision medicine, medical devices and bioengineering. Camille has a PhD from the University of Cambridge and the EMBL-European Bioinformatics Institute. Her PhD research focused on the combined analysis of various sources of high-content data to reverse engineer healthy and diseased cellular signalling networks, and the effects of drugs on these networks. Prior to that, she completed a Master’s degree in Bioengineering at the University of Brussels and a Masters in Computational Biology at the University of Cambridge.
Email: camille.terfve@mewburn.com
Our IP specialists work at all stage of the IP life cycle and provide strategic advice about patent, trade mark and registered designs, as well as any IP-related disputes and legal and commercial requirements.
Our peopleWe have an easily-accessible office in central London, as well as a number of regional offices throughout the UK and an office in Munich, Germany. We’d love to hear from you, so please get in touch.
Get in touch