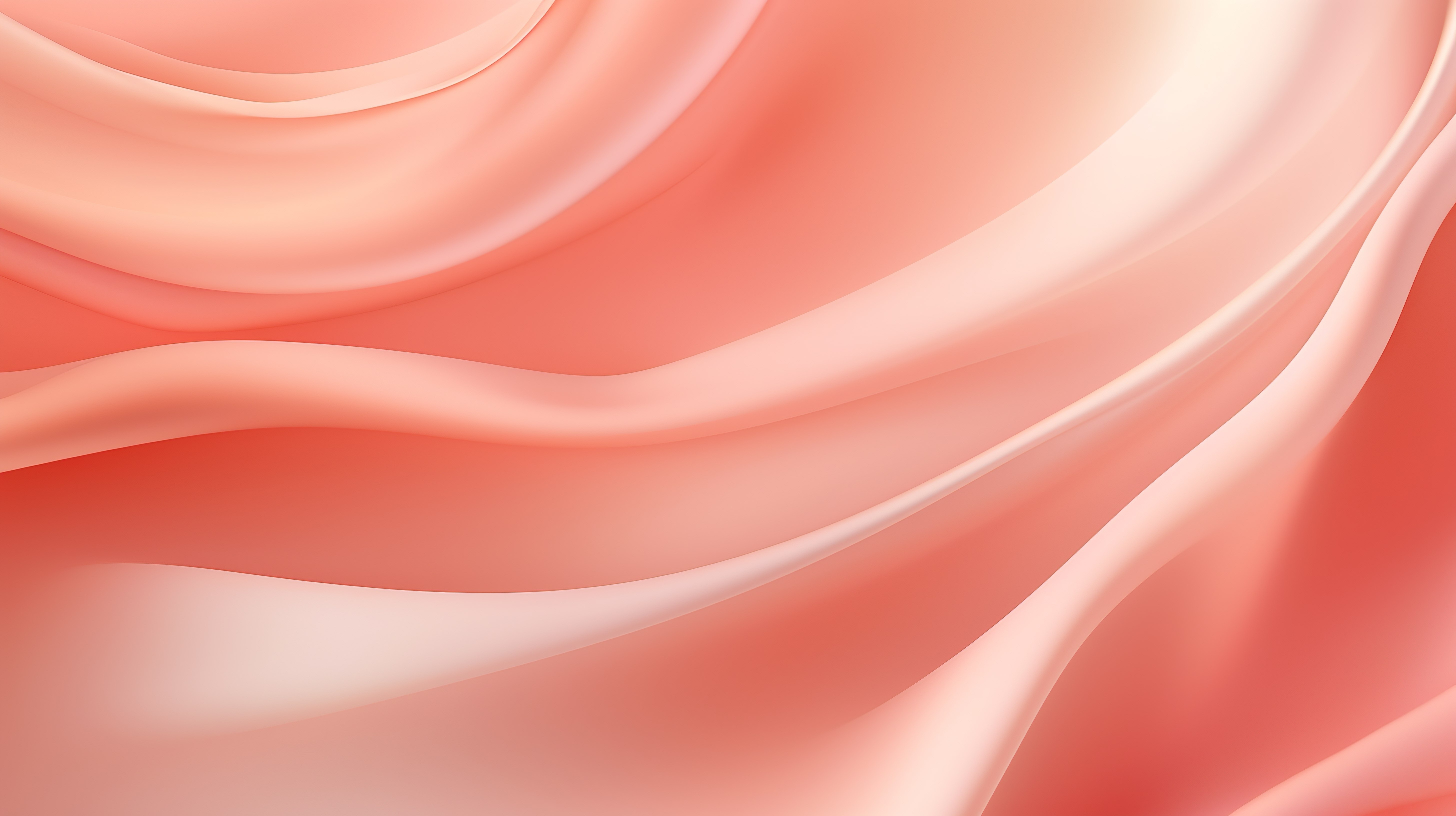
As discussed in a previous post, most types of biological or medical data that can be collected today have a high-throughput and/or high content equivalent. High-throughput assays (enabling the rapid collection of data from a large number of samples) and high-content assays (enabling the rapid collection of data about a large number of features from each sample) have dramatically changed the nature and sheer amount of data that comes out of biological and biomedical assays, and opened new possibilities that have underlined and benefited from the development of the field of bioinformatics. A few examples are discussed below.
NGS has enabled researchers to sequence countless genomes, supported by the development of tools for the analysis of such data (e.g. algorithms for base calling, sequence assembly, sequence alignment, statistical analysis of read data etc.). The data coming out of these assays has powered advances in genomics (automated annotation of genomes, genome-wide association studies, genetics of diseases), comparative genomics and computational evolutionary biology, metagenomics, etc. Knowledge of genome sequences has supported advances in our understanding of gene expression and regulation, protein structure, metabolomics etc. In the last few years, single cell sequencing technologies have enabled researchers to go beyond cell-population summary measures and look at variability and heterogeneity in populations of cells. This has resulted in invaluable insights into the aetiology of diseases such as cancer.
In the late 90’s, DNA microarrays revolutionised our ability to study gene expression and regulation by enabling the parallel quantification of thousands of transcripts, variants (e.g. splicing variants) and regulated genomic regions (e.g. through chromatin immunoprecipitation) – compared to e.g. a handful of transcripts being quantifiable in parallel by qRT-PCR. With the decreasing costs and increasing power of sequencing technologies, many of the use cases for DNA microarrays have since been replaced by sequencing equivalents (and single cell sequencing equivalents), with even increased precision and throughput.
The development of protein microarrays , and more recently advances in quantitative proteomics by mass spectrometry has made it realistic to study in parallel the expression of hundreds to thousands of proteins as well as their regulation by e.g. phosphorylation or glycosylation.
In addition to the single cell optimisation of NGS technologies, many other technologies have developed that generate data with single cell granularity. Flow cytometry has enabled scientists to look at various features of populations of cells including their size, expression of markers etc. The past decade has seen an explosion in the number of phenomenon that can be studied at the single cell level through the use of microfluidics devices that can isolate individual single cells in droplets of liquid. Due to their level of granularity, each of these methods has the ability to generate an amount of data that naturally places them in the field of application of bioinformatics.
Protein structure determination by X-ray crystallography, NMR spectroscopy and electron microscopy have also enormously benefited from the development of computational approaches. Further, the increased availability of sequence and structure information has enabled the development of structure prediction tools, protein domain analysis tools, structure and interaction modelling tools, opening new doors for rational and/or automated drug design, optimisation and repurposing, amongst other applications.
As more data of various types mentioned above became available, it became possible to study of the complex interactions between biological molecules as networks or complex systems, such as for example metabolic networks, cellular signalling networks, gene regulatory networks or protein-protein interaction networks, thereby moving from a “one or few at a time” approach to a more global and comprehensive view of biological systems.
The development of machine learning and artificial intelligence algorithms that are able to automatically analyse imaging data has already changed many aspects of biomedical imaging (e.g. automating the analysis, assembly, visualisation etc. of many imaging technologies such as x-ray, ultrasound, magnetic resonance imaging, etc.) as well as fundamental biological research (e.g. automating the high-throughput scanning of microscope and fluorescent microscope images, the behavioural analysis of model animals, etc.)
Text mining and ontologies have supported the analysis of the masses of biological and medical knowledge that has accumulated through the use of any of the above technologies. These have been essential to tasks such as annotation of data, functional genomic analyses, etc.
Combining data about medical conditions, such as e.g. the presence of a disease or subtype of disease, data about response to treatment, relapse, comorbidity, medical history, etc. can be advantageously combined with many of the other types of data mentioned above, in order to develop a better understanding of the aetiology of diseases, and to tailor therapies to patient’s molecular profiles.
Camille is a Partner and Patent Attorney at Mewburn Ellis. She does patent work in the life sciences sector, with a particular focus on bioinformatics/computational biology, precision medicine, medical devices and bioengineering. Camille has a PhD from the University of Cambridge and the EMBL-European Bioinformatics Institute. Her PhD research focused on the combined analysis of various sources of high-content data to reverse engineer healthy and diseased cellular signalling networks, and the effects of drugs on these networks. Prior to that, she completed a Master’s degree in Bioengineering at the University of Brussels and a Masters in Computational Biology at the University of Cambridge.
Email: camille.terfve@mewburn.com
Our IP specialists work at all stage of the IP life cycle and provide strategic advice about patent, trade mark and registered designs, as well as any IP-related disputes and legal and commercial requirements.
Our peopleWe have an easily-accessible office in central London, as well as a number of regional offices throughout the UK and an office in Munich, Germany. We’d love to hear from you, so please get in touch.
Get in touch