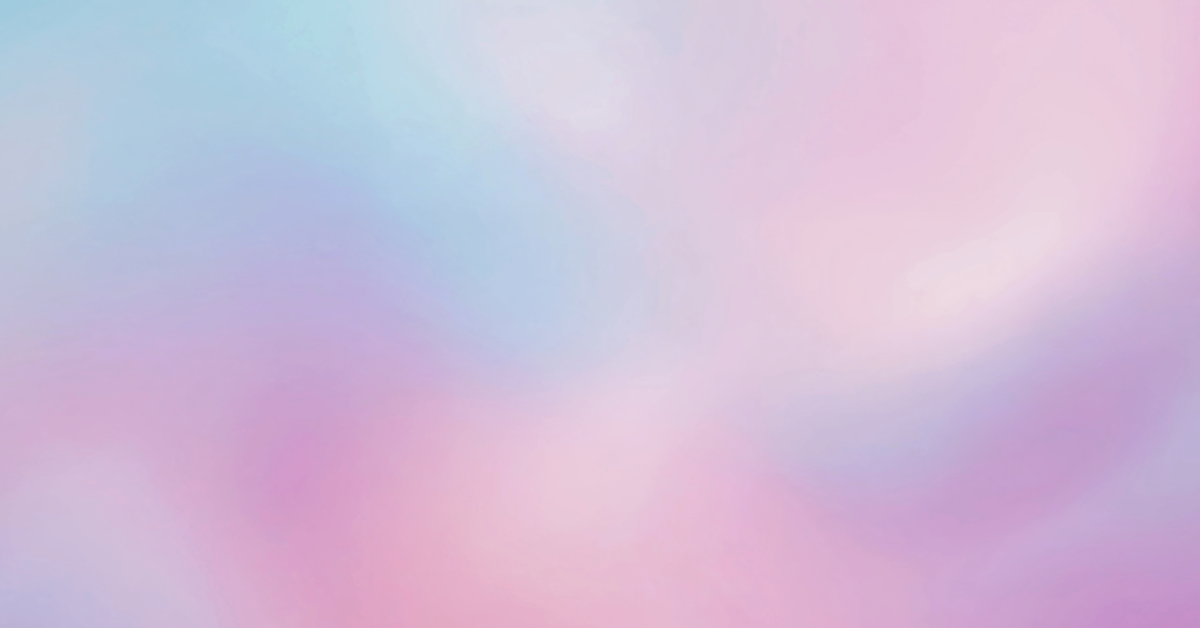
Deep learning has just made a new headline grabbing achievement: DeepMind’s latest AI-powered system AlphaFold recently demonstrated a major leap in accuracy in the prediction of protein structure from sequence (“the protein folding problem”) in the Critical Assessment of protein Structure Prediction (CASP) challenge.
Predicting the 3D conformation of a protein is hard. Proteins are very complex molecules and the number of possible configurations that can be adopted by even small proteins is mind boggling. It’s a worthwhile challenge to tackle though, since protein function and how to interfere with this are ultimately dependent on the protein structure. If you think that this does not concern you, think again: the vast majority of the drugs we use interfere with protein function.
Experimentally determining protein structure is no small feat either (and has in fact proved impossible so far for a great many proteins). As a result, much effort has been focused on in silico prediction of protein structure, fostered by initiatives such as CASP which benchmarks candidate approaches by providing a series of protein folding challenges for teams to solve.
DeepMind first entered CASP in 2018, where it already made quite an entrance by topping the leader board. DeepMind’s contribution, termed AlphaFold, was later published in Nature and the code was made freely available for research and non-commercial use.
This year, the new version of AlphaFold not only out-performed all other candidates (and its own previous version), it achieved unprecedented levels of accuracy that are in many cases similar to those achievable by experimental approaches. Of note, AlphaFold was not the only AI-powered approach at CASP this year. It was however the most successful by far (on average[1]).
The AlphaFold model has not yet been published, but DeepMind has indicated that the new version uses an attention-based neural network system that uses evolutionarily related sequences, multiple sequence alignment (MSA), and a representation of amino acid residue pairs to predict protein structure modelled as a spatial graph where residues are nodes connected by distance-dependent edges.
Reaching near experimental accuracy is a very significant line, and one that might open the door to structure determination using data that is easier to collect, or for proteins where experimental data simply could not be collected. The applications are endless both for fundamental research and for applied research. Repercussions will even reach the IP world, and the UKIPO is currently exploring (as did other IP offices such as the USPTO) the many ways in which AI is changing most fields of technology. A publication from the DeepMind team is set to follow, and I for one cannot wait to read it.
Camille is a Partner and Patent Attorney at Mewburn Ellis. She does patent work in the life sciences sector, with a particular focus on bioinformatics/computational biology, precision medicine, medical devices and bioengineering. Camille has a PhD from the University of Cambridge and the EMBL-European Bioinformatics Institute. Her PhD research focused on the combined analysis of various sources of high-content data to reverse engineer healthy and diseased cellular signalling networks, and the effects of drugs on these networks. Prior to that, she completed a Master’s degree in Bioengineering at the University of Brussels and a Masters in Computational Biology at the University of Cambridge.
Email: camille.terfve@mewburn.com
Our IP specialists work at all stage of the IP life cycle and provide strategic advice about patent, trade mark and registered designs, as well as any IP-related disputes and legal and commercial requirements.
Our peopleWe have an easily-accessible office in central London, as well as a number of regional offices throughout the UK and an office in Munich, Germany. We’d love to hear from you, so please get in touch.
Get in touch