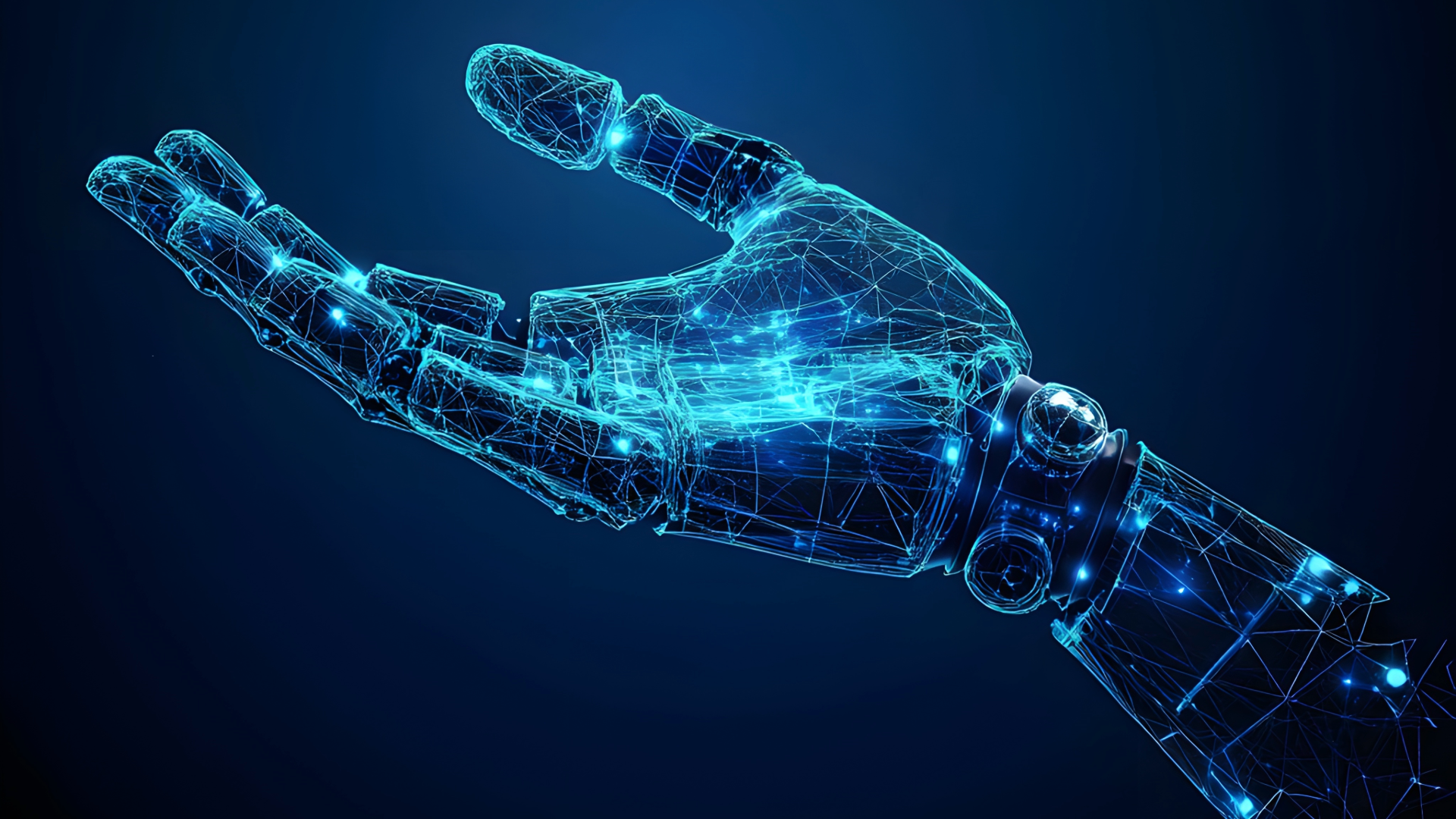
You may have come across the “animals vs. food” picture quiz on the internet. One example is Chihuahua vs. blueberry muffin:
Chihuahua or blueberry muffin?
Some of the pictures require a second look for identifying the dog or muffin. Actually describing why one picture depicts a dog and the other picture a muffin can be trickier.
The capability to distinguish objects whose visual appearance is similar is a highly specialised capability of the human brain. Attempts to replicate this capability often employ deep neural networks (DNNs) - although applications are generally more serious than sorting Chihuahuas from blueberry muffins. One application of DNNs is in self-driving cars for identifying moving objects and projecting their trajectory.
To this end, the surroundings of self-driving cars are monitored by cameras, LiDAR (which is a method for measuring distances by means of laser scanning) or other non-camera based distance sensing techniques such as radar. The individual techniques are often combined to provide redundancies.
Optical flows from the cameras or LiDAR may be analysed by a DNN in order manoeuvre the self-driving car through its environment. Evidently it is crucial that the DNN accurately and robustly identifies the surroundings of the car. However, as characteristics of DNNs are developed by training rather than being fully determined ab initio, how a given DNN performs its function may not be apparent, i.e. it may be unclear which parameters and information cause the DNN to identify a pixel pattern as a particular object. In other words, DNNs are a black box. In the example of the “Chihuahua vs. blueberry muffin” challenge, it is not generally apparent why a DNN identifies a picture as a dog and not as a muffin, even if the identification is correct.
It has been demonstrated in a recent publication that the analysis of camera videos can be severely impacted by particular patterns placed in sight of the camera. The pattern may be as small as 1% of the image captured by the camera and can be placed anywhere in the sight of the camera, for example on or close to a road sign. Such a disturbance is therefore not just a theoretical concern, but could be replicated in the real world, for example as a malevolent attack on a self-driving car or as an accidental circumstance in which a random structure in the real world happens to have a pattern capable of disturbing the DNN.
Depending on the type of the DNN, the disrupting pattern may cause complete loss of the tracked moving object, meaning that the effect of the pattern is not limited to the detection of the pattern itself, but affects the analysis of the whole image. This could be akin to becoming suddenly blind during driving. A video demonstrating such disruptions can be watched here.
Car manufacturers are understandably cautious about disclosing how self-driving capabilities are achieved (see our blog Thoughts on the transition to fully autonomous driving). However, it is reasonable to assume that much of the information gathered by cameras and LiDAR for imaging the surroundings of the car is processed by DNNs. As car manufacturers are aware of the problem of optical flow disruption, they are doubtless working on solutions. In fact, the publication mentioned above identifies certain types of DNN which are less affected by the disrupting pattern.
Besides this software fix, there is the possibility to address the pattern-induced disturbance by hardware. LiDAR and other non-camera based distance sensing techniques are not susceptible to disturbance by the above described two-dimensional patterns since these techniques - unlike cameras - do not react to colour patterns. The combination of different techniques for monitoring the surroundings of a self-driving car not only provide redundancies but also have the capability to bridge a potential weaknesses of an individual technique.
Huge amounts of work have been invested in the vision of a true self-driving car. This includes contributions not only from car manufactures, but also from software companies having expertise in DNN, and companies specializing in LiDAR and other imaging techniques.
It remains exciting to see how and when self-driving cars will be brought safely to market. This excitement of looking forward drives us at Mewburn Ellis as being the forward-looking IP firm.
Urs is a Partner and Patent Attorney at Mewburn Ellis. He helps many companies working at the frontiers of science and technology to build their IP portfolios and grow their businesses. He is genuinely enthusiastic about the different solutions these companies offer and the technical areas into which they are expanding.
Email: urs.ferber@mewburn.com
Our IP specialists work at all stage of the IP life cycle and provide strategic advice about patent, trade mark and registered designs, as well as any IP-related disputes and legal and commercial requirements.
Our peopleWe have an easily-accessible office in central London, as well as a number of regional offices throughout the UK and an office in Munich, Germany. We’d love to hear from you, so please get in touch.
Get in touch