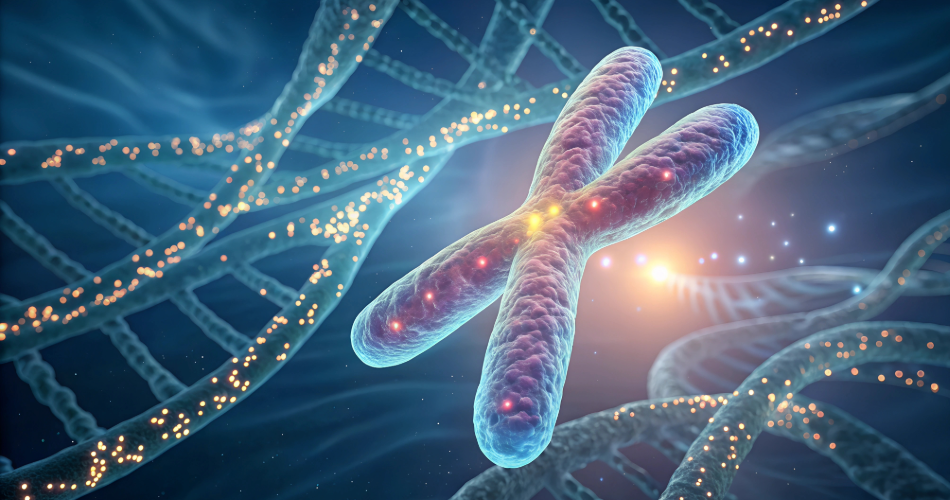
Having attended the Festival of Genomics & Biodata 2020 conference last week, it is clear that now is a good time to be working in bioinformatics1. The energy – and the investment – in the field is palpable…and the amount of data being generated, mined and shared is mind blowing. Which begs the question: are we at a stage where we have more data than we really can use, or do we not have enough data to realise the full potential of genomics?
The UK has a long history of generating genomic data – and lots of it. For example, the 100,000 genomes project led by Genomics England was completed in 2018. The 100k Genomes dataset comprise sequences from 100,000 genomes from around 85,000 NHS patients affected by a rare disease, or cancer. Additional data will be coming out of the clinic at an unprecedented scale, thanks to the NHS’s ambition to introduce whole genome sequencing (WGS) as part of routine clinical care. The NHS Genomic Medicine Service has just signed a deal with Illumina, aiming to sequence 500,000 whole genomes over the next 5 years. In the first instance, sample from patients with specific rare diseases and some cancers (all defined in the National Genomic Test Directory) will be eligible for WGS.
Staying with the theme of “let’s sequence lots of people!”, AstraZeneca’s 2 million genomes initiative aims to analyse the genome of 2 million individuals by 2026, coming from partners and AZ’s own clinical trials. The underlying goal is for the company to use the data to identify new targets and improve patient stratification and diagnostic. In order to achieve this, they have developed an internal cloud-based platform for petascale genomics. In December 2019, their platform was able to analyse 100k genomes in 80 hours, using a mostly automated workflow. The fact that AstraZeneca is focussing so much resources on obtaining such a tremendous dataset is a clear indication that big scale genomics data is a crucial pillar of modern drug discovery.
Indeed, we all know that drug discovery is an expensive and failure-prone process, and successful new drugs are painfully rare. Genomics is widely seen as one of the tools that can help solve this problem. The Open Targets initiative, a public-private partnership that uses human genetics and genomics data for systematic drug target identification and prioritisation is offering a data integration platform that combines data from 20 data sources of genetic associations, somatic mutations, drugs, pathways & systems biology, RNA expression, text mining and animal models as evidence for target identification and prioritisation. This includes data generated as part of the project such as data from a genome-scale CRISPR–Cas9 screen in 324 human cancer cell lines from 30 cancer types.
The word(s) on all the lips however is “UK Biobank”. The initiative involves the collection of rich data from a prospective cohort of 500,000 people recruited via the NHS across the UK. The data collected at the time of enrolment includes detailed lifestyle and environmental factor information, personal and family information, cognitive function information, physical measurements, blood, urine and saliva samples. Initially all patients were genotyped using a custom build Affymetrix chip array with 850k variants, and this data has already been made available. Data from whole exome sequencing of all 500k patients (led by Regeneron) will be available in 2021, and whole genome sequencing data will follow in 2022, with the first 50,000 to be completed (i.e. sequenced and variant-called – the former performed by the Wellcome Sanger Institute and the latter by Seven Bridges) by spring 20202. The data will be made available (on application) to any bona fide researcher doing research in the public interest. The project also plans to expand beyond genomics, by including imaging data for 100k people in the cohort (MRI of brain, heart and abdomen, carotid ultrasounds, full body DXA, and a repeat of the baseline assessment). Repeat imaging of all of these people will also be performed. This is an exceptionally riche dataset, of an exceptional scale.
UK Biobank is not even the only state-level initiative aiming to collect data to make the genomics health revolution a reality. The Accelerating Detection of Disease programme will carry out up to 5 million polygenic risk score assessments on volunteers. The data created will allow the evaluation of new polygenic risk scoring across millions of volunteers to see if and how we can incorporate them into smarter, more targeted clinical trials, research, and screening programmes. It will be made available to researchers from academia and industry, creating one of the largest and deepest dataset of this kind for medical and diagnostic research in the world. On the other hand, the NIHR BioResource will recruit a panel of 200,000-400,000 volunteers with rare or common disease, or healthy at the time of recruitment. Whole genome sequencing or genotyping will be performed on these participants, as well as deep phenotyping, metabolomics, and the collection of health related data and integration with medical records.
The amount of data that is being generated is clearly unprecedented. Perhaps more importantly, we are starting to see datasets that integrate multiple omics and real world data on large cohorts of individuals. Such data is extremely valuable to the research community both in industry and academia. Further, the clear push for insights to be fed back to clinical care as soon as possible is bound to give this research a boost.
It is a known fact that bioinformaticians never have enough data. It would always be nice to have a little more samples, a few more orthogonal data sources, a different control population, a different set up of co-variables, etc. Ready the above, it is easy to think that this would just be fussiness. Yet the build-up of enormous rich data sets is creating an equally enormous blind spot, and a few age-old challenges - at scale.
The central paradigm of machine learning is that your model can only ever be as good as your data. If a model has been trained on a specific population, it will have limited applicability for a different population. That creates a first blind spot. Additionally, without data on the “different population”, it is very hard to know quite how limited this applicability is. That second blind spot is dangerous because if we are not careful it can lead us to make entirely wrong predictions – possibly entirely wrong clinical decisions for people who were not sufficiently represented in the training population.
This is a problem because the vast majority of the genomic data accumulated to date comes from the USA, the UK and Iceland. Datasets like those of UK biobank or deCODE are invaluable, and we should absolutely keep collecting this data – it has already and will continue to enable life-changing innovation. The problem in fact is that the data they generate is so useful that it risks unintentionally skewing research towards tailoring the best of future healthcare to a largely white Caucasian population. Projects such as South Asia Biobank, H3Africa and others are working to fill the diversity gap, but there is still a long way to go.
Another challenge that becomes more prevalent as data accumulates is data integration and sharing. The full benefit of the data collected can only be realised if various data sets can be combined and shared. This problem is as old as bioinformatics itself, of course. However, the scale and the speed at which the data is being collected means that it is very important to act now or regret it later. Organisations such as ga4gh (Global Alliance for Genomics & Health) are working on developing frameworks and standards for genomic data sharing. This is certainly a crucial part of the solution. The problem of data integration is very dependent on the features of the data being collected. This is likely to always be a challenge, although storing the data in a way that maintains the ability to re-analyse raw data is helpful (and non-trivial considering the scale of these projects!)
Finally, collecting data on large populations in a more or less agnostic manner is unlikely to be the best way to answer a specific question, especially if that question involves a rare condition and/or comorbidity. As pointed out by Rory Collins (Chief Executive of UK Biobank), when using all 500,000 UKB participants to study the association between blood pressure and coronary heart disease (i.e. a major risk factor for a common disease), the researchers at UKB found that they still needed to follow 500k people for 10 years to see a confident linear association. Studying rarer diseases and/or less straightforward risk factors would need even larger prospective cohorts. In other words, there is still a need for hypothesis driven data collection on a less gargantuan scale. And of course, we do still need people with the time and resources to analyse that data.
As a true bioinformatician, I would have to conclude that it is extremely exciting that so many people are working to collect and share very large amounts of data…but we also do need more (other) data! Finally, I believe that to make the genomics health revolution possible, we need two things: education and public engagement. This will enable us to actively inspire the next generation to work in this field (we will need manpower to exploit all of the data that is available to its full extent), and to gain and retain the trust and support of the people for whom the research is ultimately done: patients.
1 Apparently ‘bioinformatics” is a little ‘blue collar’ and we should re-brand it as AI in biodata. I agree with Chris Wigley of Genomics England on this – if that’s the case then I’m ok being blue collar. Most ‘AI’ out there is machine learning (ML) – bioinformatics included machine learning on biodata before it became too hot to call it bioinformatics! As mentioned by Paul Agapow when asking the question “what is ML/AI?”: when talking to investors we call it ‘AI’, when talking internally we call it ‘ML’, when doing it it’s logistic regression.
2 If, like me, you are interested in the details of how these people are accomplishing this task, here are the details:
Pilot phase (also known as Vanguard project): WGS of first 50k participants of the UKB project – sequencing was recently completed by the Welcome Sanger Institute (WTSI), with analysis performed by Seven Bridges. Full completion including variant call will be available by May 2020.
A consortium was formed to sequence the other 450k, which includes the WTSI and deCODE. To meet the goal of sequencing all of these samples by 2022, the WTSI is currently sequencing 3000 whole genomes every week. To put this in perspective, the first human genome took 10 years – according to Tim Cutts of WTSI, the institute has sequenced more bases in the last 12 months than in the past 25 years put together. The analysis of these sequences will still be delivered by Seven Bridges. This is a huge endeavour in terms of logistics of sample processing (think about the sheer amount of reagents that needs to come in at the right time to sequence 3,000 genomes a week, in what was primarily built as a research institute, not a logistic centre), and in terms of data storage and analysis. Indeed, the data from the sequencers needs to be quality controlled, base called and forwarded for analysis at the same speed as it is being produced, because there is simply not enough storage capacity available to do it any other way.
In practice this means that within 24h, the WTSI does the sequencing and QC, then the unaligned files and QC reports are passed to Seven Bridges. Seven Bridges (who run their analysis for this on Google cloud / Amazon web services) have 48h to deliver the variant call. All of the data then goes to the EMBL-EBI who manages its storage. The joint variant call of all 500k genomes will be performed at the end of the project. All workflows used by Seven Bridges are encoded in open standards – when the data is released, researchers can pick up the same workflow and use it with their own data to harmonise with the UK Biobank data. According to Sinan Yavuz, SevenBridges is analysing 11k genomes per months using automated workflows that almost entirely avoid human error. Currently, all of the sequences are aligned to a single genome reference – i.e. unfortunately Seven Bridges is not using their Graph Genome approach for this data (though re-analysis using this approach would be possible).
Camille is a Partner and Patent Attorney at Mewburn Ellis. She does patent work in the life sciences sector, with a particular focus on bioinformatics/computational biology, precision medicine, medical devices and bioengineering. Camille has a PhD from the University of Cambridge and the EMBL-European Bioinformatics Institute. Her PhD research focused on the combined analysis of various sources of high-content data to reverse engineer healthy and diseased cellular signalling networks, and the effects of drugs on these networks. Prior to that, she completed a Master’s degree in Bioengineering at the University of Brussels and a Masters in Computational Biology at the University of Cambridge.
Email: camille.terfve@mewburn.com
Our IP specialists work at all stage of the IP life cycle and provide strategic advice about patent, trade mark and registered designs, as well as any IP-related disputes and legal and commercial requirements.
Our peopleWe have an easily-accessible office in central London, as well as a number of regional offices throughout the UK and an office in Munich, Germany. We’d love to hear from you, so please get in touch.
Get in touch