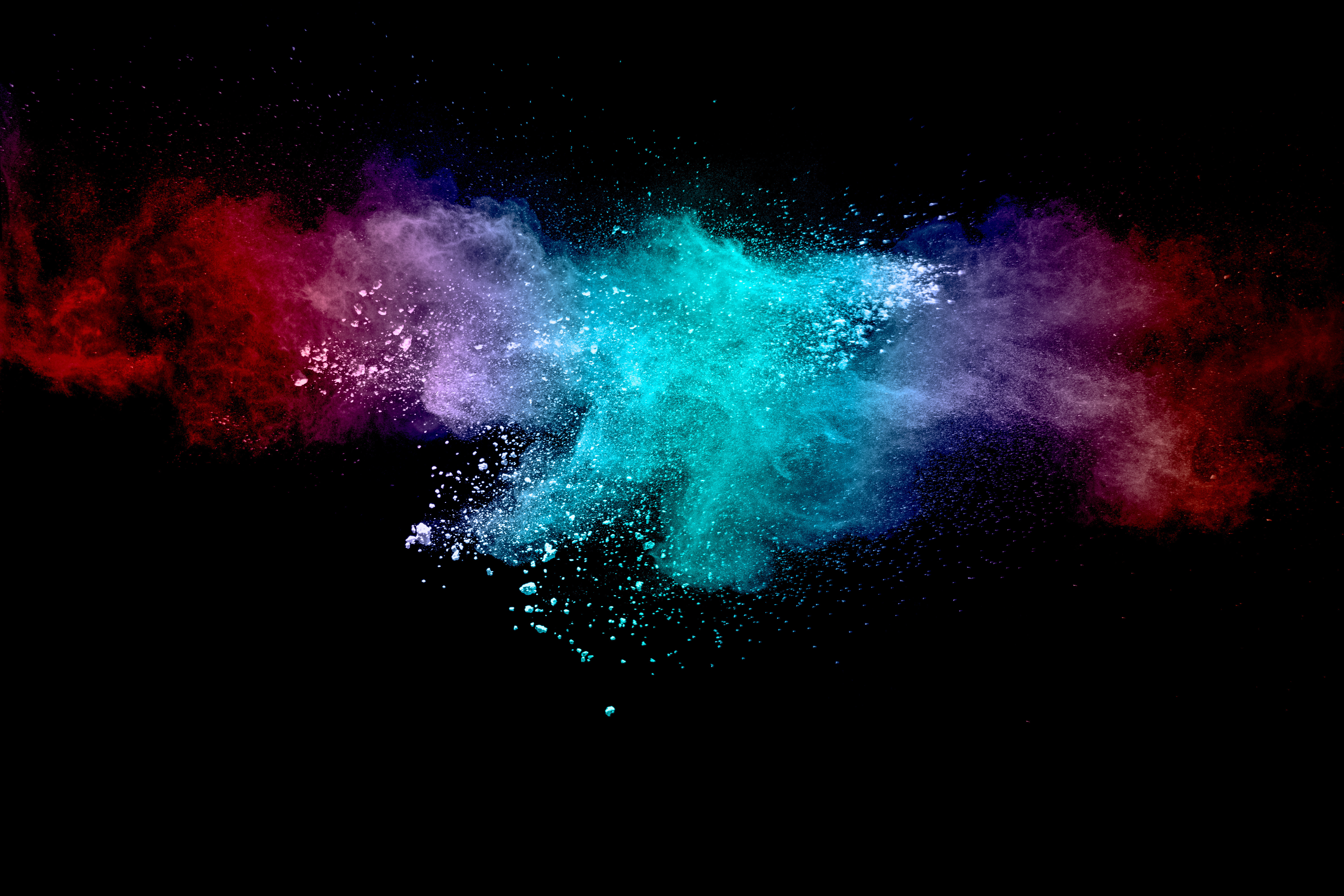
Computer simulations are solving challenges previously thought uncrackable.
Forward: features are independent pieces written for Mewburn Ellis discussing and celebrating the best of innovation and exploration from the scientific and entrepreneurial worlds.
AI can beat grandmasters at chess and outwit the world’s best at Go – but is it better than humans at coming up with new drugs? In 2016, Exscientia, an AI-powered drug discovery start-up, decided to find out.
It asked ten chemists to play a game in which they used their knowledge and experience to decide which of hundreds of chemical structures held the most promise as new medicines. Their opponent was a drug-hunting algorithm.
Twenty rounds later, the scores were totted up. The AI had beaten all but one expert.
The chemists were from pharmaceutical firm Sunovion and in 2020, a treatment candidate for obsessive compulsive disorder precision-designed by Exscientia for Sunovion’s parent company, Sumitomo Pharma, became the first AI-designed drug to enter clinical trials.
In total, Exscientia now has two owned or partially owned drug candidates in clinical trials, two in development at Sumitomo Pharma, and two further poised to go into humans for the first time this year.
It has collaborations with the likes of Sanofi, Bristol Myers Squibb, and Merck KGaA, and is working with the Bill & Melinda Gates Foundation on infectious disease targets.
The company, which started out in Dundee in 2012 with just five staff, is now headquartered in Oxford and boasts more than 400 employees. Its October 2021 floatation on the Nasdaq stock market raised $510 million, the largest initial public offering for a European biotech.
Exscientia is far from alone. According to a recent analysis by Boston Consulting Group (BCG), companies that use AI to develop drugs have a combined early-stage pipeline that equates to 50% of that of big pharma.
The 20 AI-driven drug discovery companies in the 2022 analysis, including Relay Therapeutics, Nimbus Therapeutics and Healx, together had around 160 compounds and programmes at the discovery and pre-clinical stage; this compares to the 330 or so disclosed by the top 20 pharmaceutical companies.
In a report commissioned by the Wellcome Trust, BCG states: ‘AI has the potential to materially alter the economics of innovation, allowing new medicines to be discovered for a much wider set of conditions and patient segments and by a wider membership of the research community than is possible today. If this potential can be realised, the impact on human health and health equity could be profound.’
‘I'm quietly optimistic that what Exscientia and other companies are trying to do will genuinely transform the way that we design and develop drugs,’ says Dr Dave Hallett, Exscientia’s chief scientific officer and interim CEO. ‘Within the next ten years, the majority, if not all new drugs, will be designed and developed with expert-led AI.’
Getting a new drug to market is time-consuming and expensive. With just one in ten drugs that enter clinical trials eventually making it to market, it is also fraught with risk. The entire process from discovery to approval typically takes 12 to 15 years and has an estimated average price tag of $2.5 billion, when the cost of failures is factored in.
AI has the potential to massively speed things up, cut costs and, most importantly, increase the probability of success.
For example, AI can be used to sift through large amounts of data to identify a protein or other molecule that is associated with the disease of interest and then design a drug that will bind to this target. This could be by running simulations that reduce the need for time-consuming lab tests, doing calculations on the physical properties of a compound (such as how quickly the potential drug might dissolve in the stomach or how long its effects will last), prioritising which drug candidates to investigate further or guiding the design of clinical trials.
Exscientia is using AI for all these things and more. This has enabled it to slash the number of experiments needed to make a new drug candidate and get it to the point at which it is ready for clinical trials.
Insilico Medicine has an AI-powered robotics lab that tests and validates compounds, and feeds data back into the company's end-to-end generative AI drug discovery platform
Traditionally, the industry would synthesise 2,500 to 5,000 compounds to get one that is suitable for testing in humans, Exscientia typically makes between 150 and 250. The timeline is also compressed, from four to five years to 12 to 18 months.
The process is iterative. Rather than screening hundreds of thousands or even millions of compounds in the hope of eventually hitting on one that’s the right fit, small batches of compounds are selected by the AI-led process and made, these are tested and the results used by the generative AI platform to select and make another batch of compounds. With each round of testing, the AI-platform is learning more and more about what an effective treatment with a favourable safety profile and other desired criteria may look like and so, it is hoped, getting closer and closer to that goal.
‘We’ve looked at how really talented drug discoverers have invented drugs and encoded it into Python scripts’ says Hallett. Each part of the process is still led by humans – ‘This isn’t about taking their jobs, it’s about freeing them up to be even more creative and focus on high-value adding work. While the automated AI-process runs smoothly in the background,’ explains Hallett.
The company has also applied its expertise in AI to personalised medicine, where it has shown the potential of AI to match therapies to patients who are most likely to respond to them. Applied to clinical trials, the approach could reduce drug failure rates.
In its work for the Bill & Melinda Gates Foundation, Exscientia is using AI methods to design bispecific treatment approaches for malaria – a single drug candidate that is designed to bind to two malaria-causing proteins rather than one.
‘That kind of design is several orders of magnitude harder,’ says Hallett. ‘It’s a bit like trying to find a key that fits two locks. It’s almost impossible for humans to do that rapidly, yet we’ve made progress.’
The hope is that the malaria parasite will be less likely to develop resistance to a compound that hits not one, but two, proteins that play an important role in its lifecycle.
AI’s ability to think outside the box allows it to come up with ‘weird-looking’ molecules from parts of the chemical universe that aren’t normally explored by chemists, says Dr Alex Zhavoronkov, founder and CEO of global, clinical-stage AI drug discovery company Insilico Medicine.
‘It’s an incredibly important feature and one that is allowing us to, for instance, go after “undruggable” cancer targets, so named because they cannot be easily targeted using standard drug design approaches’, says Zhavoronkov.
‘As many as 85% of all human proteins are thought to be “undruggable”. We recently partnered with Igor Stagljar’s lab at University of Toronto to develop new drugs for 15 to 20 “undruggable” targets, including KRAS, the most frequently mutated gene across all cancer types.’
Let’s return to AI’s mastery of board games for a moment. In 2016, DeepMind’s AlphaGo program triumphed over the world champion at Go, a 3,000-year-old game so complex that it had been considered to be out of the reach of computers.
DeepMind then used the knowledge it had gained from developing AlphaGo to create AlphaFold, an AI program that can predict the structure of proteins. The workhorses of the body, proteins underpin every biological process; as DeepMind puts it, ‘they carry oxygen in your body, let your eyes detect light and even make your muscles move’.
Most drugs work by binding to proteins but determining a protein’s 3D structure – and so, a binding site for a new drug – is far from straightforward. The experiments are fiddly and time-consuming, and can take years, and millions of dollars, to work out the exact shape of just one protein (if this can be determined at all).
AlphaFold, in contrast, can predict the structure of a protein almost instantly and, today, a database that contains the structures of more than 200 million proteins, including all of those in the human body, is freely available to scientists.
Last year [2023], Zhavoronkov was part of an international team of scientists that demonstrated the first successful use of AlphaFold in coming up with a ‘hit’ – a promising drug.
First, one of Insilico’s own programs sifted through millions of pieces of data, such as patents, publications, grants, and clinical trials, to identify a protein that could be targeted.
AlphaFold then determined the structure of the protein. Using that structure as a starting place, Insilico’s generative AI chemistry engine, Chemistry42, designed an in silico a drug capable of binding to that structure.
In February 2022, Insilico became the first company to take a drug with both an AI-discovered target and an AI-generated design (a treatment for the lung disease idiopathic pulmonary fibrosis, IPF) into Phase II clinical trials with patients.
‘Everyone is waiting to see the first AI drug cross the finish line,’ says Zhavoronkov. ‘As this drug for IPF advances, we are in the critical final stages of demonstrating that this method of finding and developing new drugs works – and it can do the work faster, better, and cheaper than ever imagined. […]The success of this drug would mean that not only patients suffering from IPF, but from other diseases, can have new hope that AI can speed new cures.’ The drug is one of five that in Insilico has in clinical trials – and one of 31 in its pipeline.
A lot has changed since Zhavoronkov launched the company a decade ago.
He says: ‘The initial reaction from the pharma industry was scepticism. Despite the terrible track record of traditional drug discovery, the industry was not originally receptive to deep learning. Now, many pharma companies are eager to license AI-designed molecules, even at earlier stages, and many pharma companies, like Sanofi, are embracing an AI-first approach.’ Indeed, the company has since concluded a deal worth $1.2 billion with Sanofi.
‘I look at AI in the same way as I look at a small child, in the sense that when you are born, you don’t know everything, you have to learn your way in the world,’ says Hallett. ‘And I think that’s where AI is – there’s a huge amount still to come.’
Written By Fiona MacRae
Camille Terfve, Partner and Patent Attorney at Mewburn Ellis, comments:
"Computational biology and chemistry is an essential part of modern drug discovery and this is not about to change. With improved AI methods coming out almost daily, the possibilities are hard to imagine even for someone like me who literally spends every day looking at new technology that leverages ML/AI in the biotech/pharma space. From an IP perspective one of the challenges is that the pace of this innovation is far greater than the pace of change of the patent system. However, in our bioinformatics team we deal with this day-in, day-out and have tremendous success getting valuable protection in this space. It’s a fun, fascinating area of IP to work in!"
Camille is a Partner and Patent Attorney at Mewburn Ellis. She does patent work in the life sciences sector, with a particular focus on bioinformatics/computational biology, precision medicine, medical devices and bioengineering. Camille has a PhD from the University of Cambridge and the EMBL-European Bioinformatics Institute. Her PhD research focused on the combined analysis of various sources of high-content data to reverse engineer healthy and diseased cellular signalling networks, and the effects of drugs on these networks. Prior to that, she completed a Master’s degree in Bioengineering at the University of Brussels and a Masters in Computational Biology at the University of Cambridge.
Email: camille.terfve@mewburn.com
Our IP specialists work at all stage of the IP life cycle and provide strategic advice about patent, trade mark and registered designs, as well as any IP-related disputes and legal and commercial requirements.
Our peopleWe have an easily-accessible office in central London, as well as a number of regional offices throughout the UK and an office in Munich, Germany. We’d love to hear from you, so please get in touch.
Get in touch