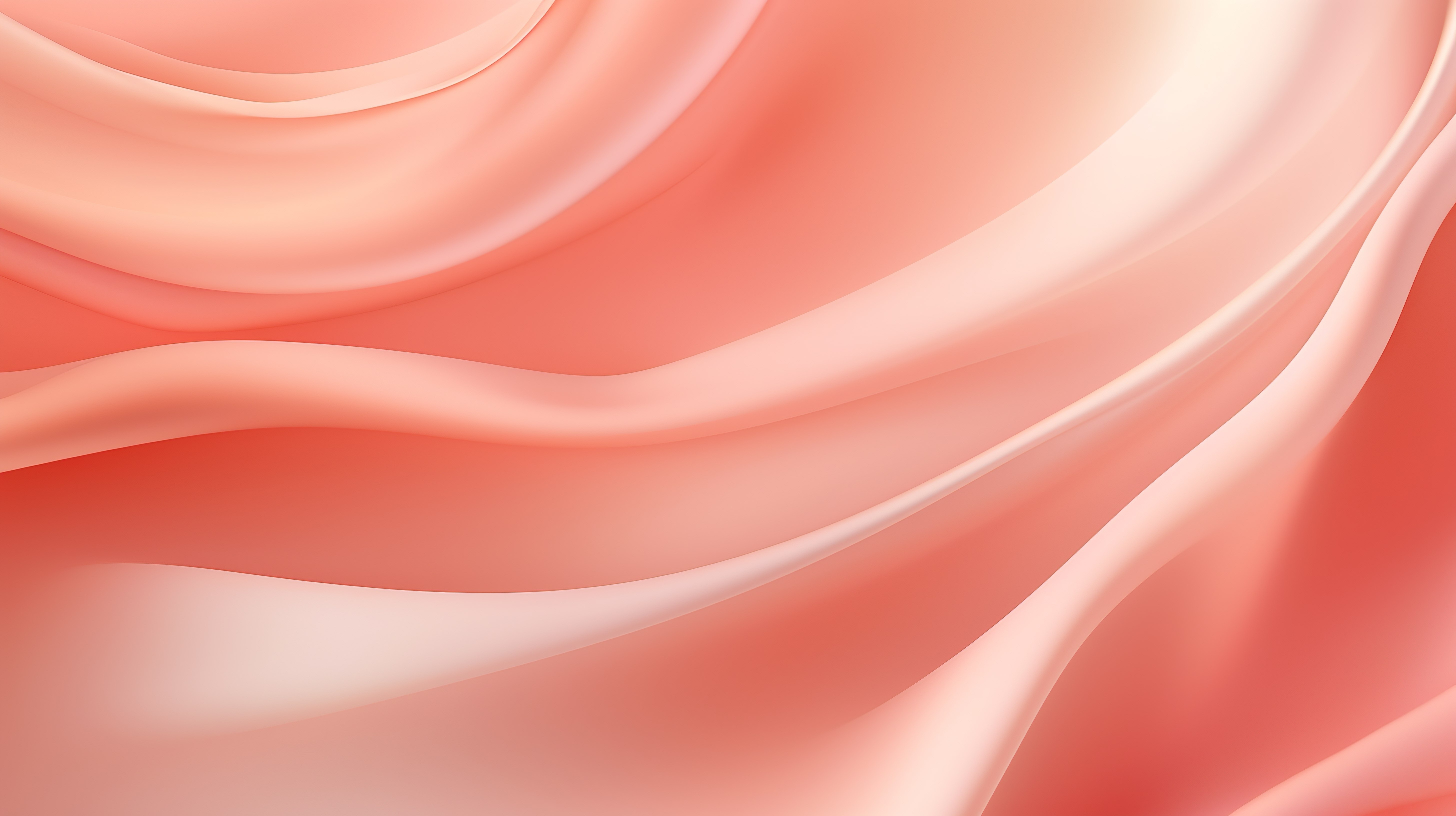
There is one obvious way in which computational biology is showing its strengths in the current climate: computational biologists can still work even during the confinement. And it’s a good thing too, because they are doing some crucial work to support decision makers, care providers and ultimately all of us to fight the Coronavirus (COVID-19) epidemic. Read on for a few highlights of this work…
Disease dynamics modelling rarely gets a chance to be in the spotlight. This wrong has been righted. It is very difficult to intuitively comprehend the features of a phenomenon like an epidemic. Many factors interact to determine the number of cases at any one time, and most of us really cannot fully grasp what “exponential growth” means until we see some numbers. The presence of a few infected people outside of China did not feel like something to worry about. We then experienced first-hand the impact of a few simple parameters like the length of incubation period, reproduction number (a measure of “contagiousness”) and infectious period of this particular virus, in a world that is more connected than ever. That is exactly what disease dynamics modelling can do: help us understand the impact of parameters that govern the spread of a disease, and the effect that interventions can have in controlling this spread.
An example of such work is the study by the Imperial College COVID-19 Response Team, which modelled the likely impact of two non-pharmaceutical strategies: (a) mitigation (a 3 months long implementation of measures ranging from case isolation alone to a combination of case isolation, home quarantine and social distancing of the over 70s, with the aim of slowing but not necessarily stopping entirely the spread of the disease), and (b) suppression (a 5 months long implementation of stricter measures such as a combination of case isolation, household quarantine and general social distancing, with the aim of reversing epidemic growth). The authors found that even in the most drastic implementation, mitigation would not prevent our health system from being overwhelmed repeatedly, and would lead to hundreds of thousands of deaths. This realisation has in part led us to the situation that we are in now: implementing a combination of social distancing of the entire population, home isolation of cases and household quarantine of their family members. However, the authors also predicted that transmission will quickly rebound if the interventions are relaxed. In other words, we may be in this situation for the long haul.
As mentioned above, non-pharmaceutical interventions are only likely to take us so far. Ultimately we will likely need drugs and/or a vaccine to win this fight. Computational biology can help here too.
Finding a new drug and bringing it to market is hard, expensive, and time consuming. It often takes 10-15 years for a drug to go from initial discovery to market, and that’s only for those that actually make it to market since lots of candidates have to be abandoned along the way. It goes without saying that we do not have that time. Over the last few years, fascinating computational approaches have been developed to help speed up and streamline this process. For example, many data-driven drug repurposing (also referred to as drug repositioning) approaches have been proposed with the aim of identifying diseases, conditions or groups of patients that could be treated with existing drugs not previously known for this indication. This has the benefit of skipping some of the 10-15 years development pipeline. Other work has focussed on computational drug design, for example through in silico evolution of drug candidates with desired properties. A British company that specialises in AI-powered drug design (spun off by some of the authors of this paper) has recently announced that they would be applying their expertise to screen a large library of drugs to identify compounds that could interfere with the replication or entry into human cells of Sars-CoV-2 (the virus behind the COVID-19 epidemic). If they are successful, this could arm us with new means to fight the virus in as little as 12 months (depending on many factors including how clinical trials would be necessary and how we are able to manufacture the new drug).
Computational biology can also help with the design of a vaccine for Sars-CoV-2. As explained in this review, mathematical modelling of viral evolution can help us understand how a virus evolves to escape the immune system. We can then use this information to be “one step ahead” of the virus in designing a vaccine. Researchers across the world are gathering genetic information about Sars-CoV-2 as it spreads, building the data set that is needed for us to better understand how this virus changes. This will be a crucial piece of information for any vaccine that is being developed now to protect us against the virus that we will be facing by the time a vaccine is ready for mass production.
We are living through unprecedented times, at least when considering the timescale of human memory. However, we are also equipped with more biological data than ever, and more people, enthusiasm and expertise than ever to analyse it. In the meantime, if you get (understandably) frustrated about working from your kitchen and spending your holidays in your living room, here are my suggestions:
Camille is a Partner and Patent Attorney at Mewburn Ellis. She does patent work in the life sciences sector, with a particular focus on bioinformatics/computational biology, precision medicine, medical devices and bioengineering. Camille has a PhD from the University of Cambridge and the EMBL-European Bioinformatics Institute. Her PhD research focused on the combined analysis of various sources of high-content data to reverse engineer healthy and diseased cellular signalling networks, and the effects of drugs on these networks. Prior to that, she completed a Master’s degree in Bioengineering at the University of Brussels and a Masters in Computational Biology at the University of Cambridge.
Email: camille.terfve@mewburn.com
Our IP specialists work at all stage of the IP life cycle and provide strategic advice about patent, trade mark and registered designs, as well as any IP-related disputes and legal and commercial requirements.
Our peopleWe have an easily-accessible office in central London, as well as a number of regional offices throughout the UK and an office in Munich, Germany. We’d love to hear from you, so please get in touch.
Get in touch