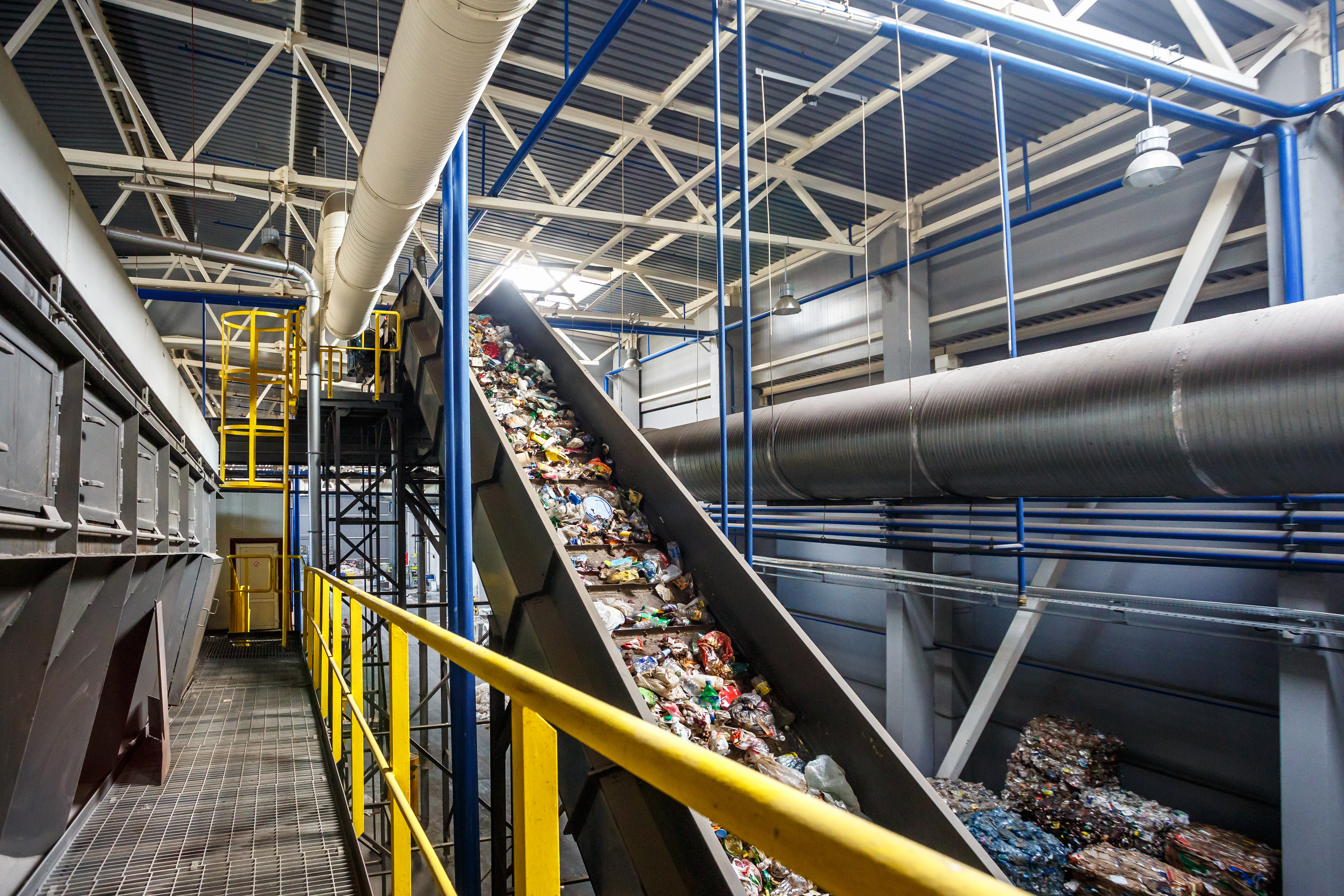
Research into new materials with desirable properties has been one of the most long-standing aspects of scientific development. For much of human existence, this always relied on pure experimentation. Now with advancements in computational methodologies, the rapidly emerging field of materials informatics offers routes to materials through machine learning as a means of rapid scoping through thousands of candidates and identifying desirables in a very short time span.
Traditionally, new materials have mostly been discovered by a “cook and look” approach - given a starting material, one decides to alter it to prepare a product in the hope of improving the target property. To synthesise a series of materials, this is both time- and resource-consuming. While there are also isolated cases in which desirable materials have been discovered by complete accident, such as Teflon and safety glass, luck-based approaches clearly not reliable.
Enter materials informatics: at its broadest definition, materials informatics is the use of data and machine learning methods in the field of materials science to gain insights into various scientific, processing or business aspects.
Firstly, from a scientific aspect, materials informatics assists researchers in predicting the properties of materials when given different input data, such as composition or structure, so that valuable candidates can be produced from a machine learning model. Secondly, from a processing aspect, rapid predictions made from large databases for material properties significantly reduces the time and effort spent by researchers on experimentation and provides results to help them decide their next steps in development. Finally, from a business aspect, materials informatics can help inform product development decisions and centralises the collective scientific (domain) knowledge within a company.
Although computational approaches, such as density functional theory (DFT), to simulate properties exist, the drawbacks of DFT include slow calculation speeds and the inability to describe disordered structures and f-orbitals. In contrast, materials informatics rapidly predicts properties, rather than having to slowly calculate them. This diminishes the reliance of luck in materials discovery – when provided with enough data, a model can provide a very accurate prediction for the researcher.
Materials informatics utilises machine learning to optimise the performance of tasks from past experience by taking input parameters and predicting output properties. For materials discovery, three main aspects are required: data, descriptors and algorithms.
As a prerequisite, there needs to be a sufficiently large amount of high-quality input data related to the problem under study. A lot of data is usually locked away in journals under various formatting and can generally be hard to extract efficiently. For this reason, databases such as the Materials Genome Initiative and the MPDS, contain information on numerous properties of known materials to provide easy access for researchers.
Descriptors, or features, are the representations or characteristics of materials. For example, this may be elemental properties, such as the ionic radius and group in the periodic table, which may be used as features to model, for instance, formation energies. Descriptors may be either numerical (e.g. atomic number) or categorical (e.g. crystal structure). It is generally useful to select suitable descriptors which have a well-known correlation between the target property and the other properties.
Algorithms govern the way that the machine learning model interprets data and should be tailored depending on the problem to be solved. For example, linear regression may be useful if there is linear scaling between two properties, like bulk modulus and hardness. More complicated algorithms include the random forest, which builds decision trees using rules to progressively narrow the conditions to generate predictions, and support vector regressions, which effectively separates data points in different clusters to capture non-obvious or non-linear effects between variables in the dataset.
Upon establishing these aspects, machine learning can make predictions. Typically in materials informatics, supervised learning is used, which aims to use a dataset containing input variables (e.g. structure) and corresponding output variables (e.g. property) to learn the mapping function from the input to the output as a means of training the model to optimise its performance. The main goal of the supervised learning approach is to ensure accuracy of predictions once it has been trained on the dataset.
The use of materials informatics to scope new desirable materials has seen success in recent years. One notable instance involved finding new superhard materials (materials with a hardness of over 40 gigapascals when measured according to the Vickers hardness test). These materials are used in abrasives and cutting tools, but existing materials required extreme synthetic conditions, or comprise very rare elements, such as rhenium and osmium.
To scope for new and practical superhard materials, US researchers in 2018 developed a machine learning algorithm to screen a database of over 100,000 materials. As the target property hardness is complex and not always disclosed as data, they used 5000 data points from DFT data predicting bulk and shear moduli as suitable correlating descriptors to build and train their machine learning model. Using their model, they were able to predict bulk and shear moduli of all the compounds in the database and, out of all those compounds, they identified two superhard materials which can be made at significantly reduced cost. Without materials informatics, the new superhard materials might only be achieved through lengthy trial-and-error.
Similarly, Citrine Informatics, a leading company in the materials informatics field, has long been involved in accelerating materials development processes using its technology. In one case, they discovered two new thermoelectric materials which would not have been obvious with intuition alone. Their approach involved the collation of electrical and thermal conductivities, Seebeck coefficients and band gaps of known thermoelectrics and the development of a set of descriptors to characterise the materials that would act as inputs to the model. To encourage its use in materials discovery, the model has since been made publicly available as a web app.
It is always preferable for researchers to understand the mechanistic reasoning or chemical origins behind existence of the properties of the new materials to rationalise the structure-property relationship, but the black-box nature of materials informatics struggles to provide the most suitable interpretability for researchers. Recently, the concept of attention-based learning is used as a way of providing interpretability to the model to visually show which descriptors are responsible for structure-property relationships.
It is important to note that, ultimately, materials informatics is a useful tool for researchers to use when discovering materials. As it will always rely on the input of data and expert knowledge, it will never be able to replace scientists. Nevertheless, this gives good reason to exploit what machines are good at. While they have good “chemical intuition” to provide suggestions for materials, at the end of the today, a human will make the final decision.
Material informatics involves computer-implemented or software-based methods, and in particular the processing of data using machine-learning methods. Contrary to widespread belief, such computer-implemented methods can be patented at the European Patent Office (EPO) in certain circumstances. In fact, the EPO’s Guidelines for Examination explicitly state that artificial intelligence and machine learning processes can be patentable at the EPO. This means that patent protection may be possible for material informatics processes or methods for discovering new materials.
To assess the patentability of such computer-implemented inventions, the EPO uses a very specific approach; to be inventive and thus patentable, the claimed invention must include at least one feature which (i) is new; (ii) is not obvious; and (iii) contributes to the technical character of the invention. For a new and non-obvious feature to contribute to the technical character, it must either be (a) technical when taken in isolation, or (b) non-technical when taken in isolation, but it contributes to a technical effect serving a technical purpose in the context of the invention. Some more detailed information about the EPO’s approach to assessing the patentability of computer-implemented methods in general can be found here, and our full guide to patenting computer-implemented inventions in the field of bioinformatics (which are assessed in a similar way to material informatics inventions) can be found here.
With regards to the materials themselves which result from a materials informatics process, they are in principle patentable just like any other new and non-obvious material.
In his TEDx talk, Dr. Taylor Sparks, a Professor of Materials Science and Engineering from the University of Utah, mentioned the 14 Grand Challenges for Engineering in the 21st Century, a series of technological challenges to be achieved for the purposes of progressing as a species. He identified that over half of these, such as making solar energy economical and providing methods of carbon capture, will inevitably involve the development of new materials.
It is therefore hoped that the road to materials discovery can be massively accelerated with the use of materials informatics. Businesses and research groups can scope for new materials dramatically faster than ever before at a fraction of the cost. While the traditional researcher would face enormous possibilities and have a one-at-a-time approach for each one, it has now been proven that desirable materials can be identified from a massive database in 30 seconds on an ordinary desktop computer.
This blog was co-authored by Nathan Zhang and Lucy Coe.
Lucy Coe is an Associate and Patent Attorney at Mewburn Ellis. She works primarily in the computer software, electrical engineering, transport, and mechanical engineering sectors. She is involved with all stages of the patent process, particularly in the drafting and prosecuting of applications in the UK and at the EPO. Among others, her areas of expertise include bioinformatics and computer implemented inventions.
Nathan is a patent attorney working in our chemistry team. Nathan has a Masters degree in chemistry (MChem) from the University of Oxford. His undergraduate research project focused on the synthesis of zirconium complexes for polymerisation catalysis. During his undergraduate studies at Oxford, Nathan developed an electrochemical method of surface-initiated polymerisation as part of a summer project. He also completed a short internship studying hydrogen thermal batteries at Stanford University in California, USA.
Email: nathan.zhang@mewburn.com
Our IP specialists work at all stage of the IP life cycle and provide strategic advice about patent, trade mark and registered designs, as well as any IP-related disputes and legal and commercial requirements.
Our peopleWe have an easily-accessible office in central London, as well as a number of regional offices throughout the UK and an office in Munich, Germany. We’d love to hear from you, so please get in touch.
Get in touch