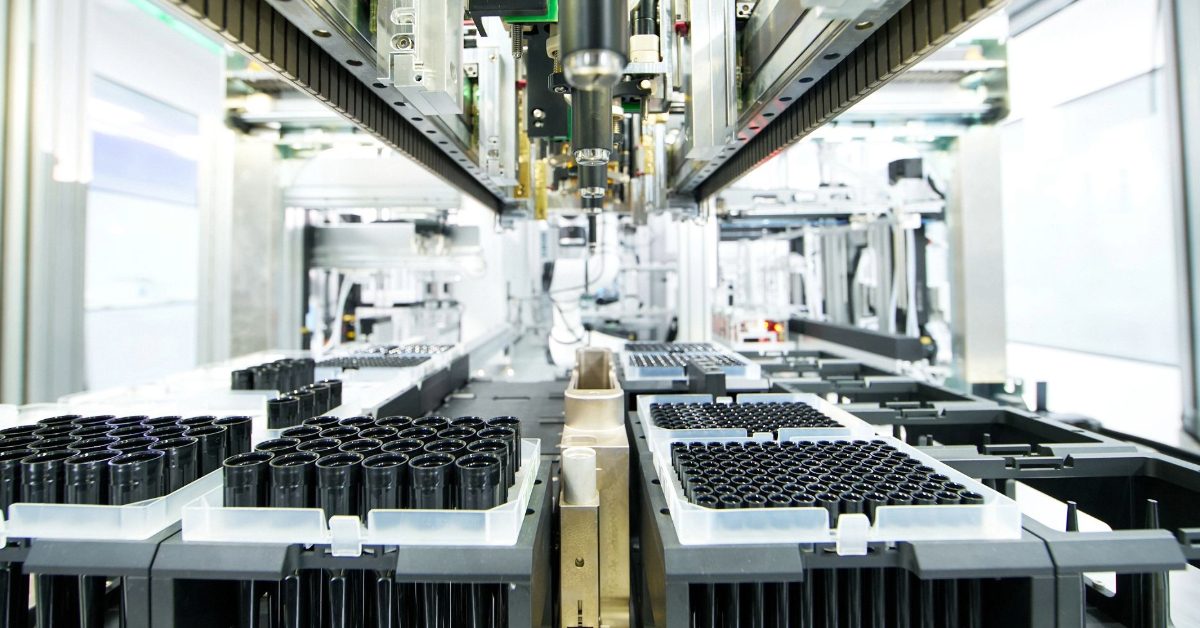
When most of us hear the term motion capture, we tend to think of special effects in films and actors rolling around in suits covered in ping-pong balls. However, two recent publications in Nature Medicine suggest that the application of motion capture can extend outside the field of CGI (computer generated imagery) and into the field of medical technology.
Friedreichʼs ataxia (FA) is caused by a variant of the Frataxin (FXN) gene, leading to its downregulation and progressively impaired cardiac and neurological function. FA affects about 1 in every 50,000 people in the US.
Duchenne muscular dystrophy (DMD) is a genetic disorder characterized by progressive muscle degeneration and weakness due to the alterations of a protein called dystrophin that helps keep muscle cells intact. DMD affects about 1 in every 100,000 people in Europe and North America.
Both conditions result in an effect on the patient’s movement. FA produces unsteady, awkward movements and a loss of sensation due to nerve injury that develops as the disease progresses. DMD results in progressive weakness in the muscles, which can begin as difficulty in jumping, running, and walking in paediatric patients and progressively worsen until the cardiac and pulmonary muscles are affected as well.
The progression of these two diseases is usually tracked through intensive testing in a clinical setting and the assessments are usually performed by the clinician by eye.
These two diseases were the subject of two recent studies. The DMD-focused study was a combined effort between Imperial College London, Great Ormond Street, and University College London, whereas the FA-focused study was led by teams at Imperial College London, University College London, and the MRC London Institute of Medical Sciences.
Both studies focused on the role that motion capture could play in the detection and monitoring of the respective diseases. The studies used different motion capture suits; however, each suit included 17 wireless inertial measurement unit sensors (motion sensors) positioned at various locations around the body. According to the DMD study, the sensors were positioned at the head, shoulders, upper arms, forearms, hands, sternum, pelvis, thighs, shanks and feet of the patients.
Once the subjects had donned their motion capture suits, they were asked to perform a variety of standardised clinical assessments (such as a 6-minute walk test in the case of the DMD study) as well as going about their daily activities. The resulting dataset is vast and tracks the relative positions of all 17 points on the subject’s body as they move around.
The results obtained from the motion capture suits have been termed “movement fingerprints”.
Much like fingerprints themselves, human movement behaviour is highly variable and complex. Previously, clinical assessments of these conditions have been done by eye. For such a complex task, it is often nearly impossible to judge small variations between assessments in order to accurately judge the progression of the conditions over time. Digital measurement techniques have been used before now, but only as a means of replicating the human assessment in a digital form.
The two studies have sought to overcome these limitations through the use of an Artificial Intelligence (AI) framework for analysing the movement fingerprints captured by the motion capture suits.
Co-author of both studies Professor Richard Festenstein, from the MRC London Institute of Medical Sciences and Department of Brain Sciences at Imperial College London said: “Patients and families often want to know how their disease is progressing, and motion capture technology combined with AI could help to provide this information. We’re hoping that this research has the potential to transform clinical trials in rare movement disorders, as well as improve diagnosis and monitoring for patients above human performance levels.”
In both studies, all of the data from the motion sensors was collected and fed into the AI technology to create individual avatars of each patient and analyse their movements. This vast dataset together with a powerful computing tool allowed researchers to define key movement fingerprints seen in children with DMD as well as in adults with FA. Many of the subtle movement patterns recognised by the AI framework had not been described clinically before in respect of either DMD or FA.
This new way of analysing full-body movement measurements provides clinical teams with clear disease markers and disease progression predictions. The studies were able to produce 6-month disease progression predictions that were significantly more accurate compared to current gold-standard assessments.
Disease markers and disease progression predictions are invaluable tools during clinical trials to measure the benefits of new treatments. The improved precision not only improves the efficiency of clinical trials, but also means that patients can access new therapies quicker and can also help to dose drugs more precisely.
As mentioned above, both DMD and FA are relatively rare conditions. One of the most significant challenges when researching rare conditions is the lack of candidates for performing clinical trials.
However, not only can this new motion capture and AI framework help researchers conduct clinical trials of conditions that affect movement more quickly and more accurately, but it can also reduce the number of candidates needed to obtain accurate results from a clinical trial.
In the DMD study, researchers showed that this innovative technology could reduce the number of children required to detect if a treatment was working to a quarter of the number required with current assessment methods. Similarly, in the FA study, the researchers showed that they could achieve the same precision with a sample size of 10 patients instead of over 160.
This AI technology is especially powerful when studying rare diseases when patient populations are smaller. In addition, the technology makes it possible to study patients across life-changing disease events such as loss of ambulation whereas current clinical trials target either ambulant or non-ambulant patient cohorts.
The researchers hope that as well as using the technology to monitor patients in clinical trials, it could also one day be used to monitor or diagnose a range of common diseases that affect movement behaviour such as dementia, stroke and orthopaedic conditions.
It is always exciting to see developments such as these in fields that have been relying on the same diagnostic and monitoring tools for a long time. It will be interesting to see if these concepts can be implemented across a wider range of conditions in the future.
Callum has experience in the drafting and prosecution of patent applications in the UK and at the EPO. He has worked with a range of subject matters including medical devices, mechanical engineering, software and consumer products.
Email: Callum.Anderson@mewburn.com
Our IP specialists work at all stage of the IP life cycle and provide strategic advice about patent, trade mark and registered designs, as well as any IP-related disputes and legal and commercial requirements.
Our peopleWe have an easily-accessible office in central London, as well as a number of regional offices throughout the UK and an office in Munich, Germany. We’d love to hear from you, so please get in touch.
Get in touch